MAX PLANCK 연구소 2022년 상반기 Pose Estimation 논문 요약
- -
Pose Estimation 분야 논문들을 보다 보니,
Max Planck(막스플랭크) 연구소 논문들이 유독 많고,
올해 나온 논문들만 벌써 24개는 되네요!
그래서 오늘은 Pose Estimation 분야에서 주도적인 연구를 이끌어가고 있는 연구소인
MAX PLANCK의 2022년 상반기 논문들 중 Pose Estimation관련 논문들을 정리해보겠습니다.
(2022-07-06 기준)
오늘은 간략하게 살펴볼 거라 디테일이 궁금하신 분들은 해당 논문을 직접 확인하시는 게 좋을 것 같습니다.
이제 따라오시죠!
자료 출처는 아래의 두 군데인데, 저는 아래 부분 (google scholar) 기준으로 설명하겠습니다.
https://ps.is.mpg.de/publications/
Max Planck Institute for Intelligent Systems
The Max Planck Institute for Intelligent Systems has campuses in Stuttgart and Tübingen. The institute combines – within one center – theory, software, and hardware expertise in the research field of intelligent systems. The Tübingen campus focuses o
is.mpg.de
https://scholar.google.com/citations?hl=ko&user=6NjbexEAAAAJ&view_op=list_works&sortby=pubdate
Michael J. Black
Max Planck Institute for Intelligent Systems - 인용 횟수 61,015번 - Computer Vision - Computer Graphics - Machine Learning - Virtual Humans - Optical Flow
scholar.google.com
크게 6가지 정도의 분야가 있습니다.
1) Body
2) Whole Body
3) Hand
4) Head
5) Face
6) 기타
하나씩 살펴보시죠.
한 글로 다 풀려고 했으나 너무 글이 길어져서, 이번 글에서는 Body 부분만 다루고, 나머지는 조만간 새 글로 작성할 예정입니다.
1. Body
1-1) Icon: Implicit clothed humans obtained from normals
CVPR 2022에 소개된 논문입니다.
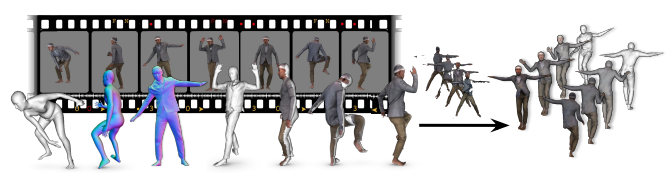
단안 이미지에서 옷을 포함한 mesh를 추출하는 작업입니다.
(View point가 하나인 이미지를 단안 이미지라 합니다. 일반적으로 폰으로 사진을 찍는다던지 하는 케이스이고,
이에 반대되는 개념으로는 여러 대의 카메라를 두고 싱크를 맞춰서 촬영하는 multi-view 이미지가 있습니다.)
이와 같은 모델은 PIFu, PIFuHD 등이 있었는데, 일상 이미지에서 추론해봤을 때 형편없는 결과였습니다.
ICON 입력값 : segmented clothed human image + SMPL
(SMPL : 옷을 입은 사람의 3D Body, ICON은 SMPL-X와도 호환 가능하다고 합니다.)
사람의 옷을 segmentation 하는 건 Self-Correction-Human-Parsing 같은 모델을 사용한 것 같습니다.
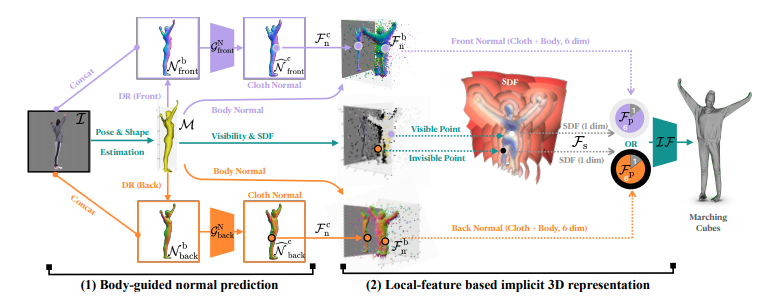
front(카메라로 찍은 방향)와 back을 병렬로 추론하고, 옷 특징을 추출하고, concat해서 합치고 있습니다.
code를 보면, colab으로도 제공하고 있습니다.
1-2) Accurate 3D Body Shape Regression Using Metric and Semantic Attributes
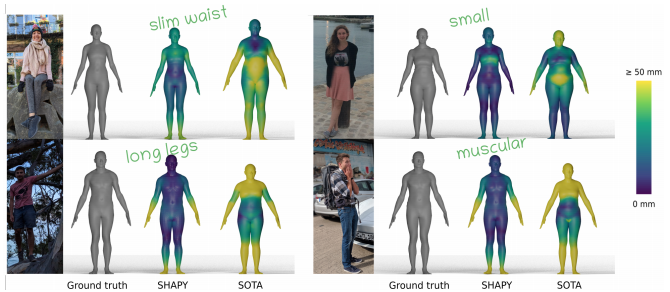
요즘 언어와 비전을 섞는 모델들이 굉장히 많은데요.
Pose Estimation 쪽에서도 해당 주제를 다룬 재밌는 논문이 나왔습니다.
일반적으로 model-based 방식에서, 3D Pose(Mesh)를 추정할 때, Pose와 shape을 추정하게 되는데요.
(반대인 model-free 방식에서는 SMPL과 같은 모델을 사용하지 않고 바로 vertex를 추정합니다.)
여기서 shape을 추정할 때, semantic attributes(의미있는 속성들, 언어적 속성)을 이용해서 보정함으로써 더 현실에 가까운 shape을 추정하도록 하는 방법입니다.
위의 이미지를 보면, SOTA 방법들에 비해, "얇은 허리, 긴 다리, 근육질의" 같은 속성들이 추가된 SHAPY 모델의 결과가 훨씬 GT에 가까운 걸 볼 수 있습니다.
이 논문에서 지적하는 것처럼, 현재 대부분의 3D Pose Estimation은 Shape보다는 Pose에 중점을 맞추고 있습니다.
https://youtu.be/7TzXrL4eV9g
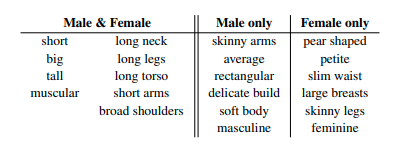
언어 속성 수치는 아마존 크라우드소싱을 통해 데이터를 수집했고,
각 속성별 1점부터 5점까지(매우 동의하지 않음 ~ 매우 동의함)의 값으로 평가하도록 했습니다.
Attributes and 3D Shape
Attribute와 3D Shape 매핑을 위해 몇 가지 방법들을 사용했습니다.
1) Attributes to Shape (A2S) : 언어 속성 score에서 shape(beta)을 예측하도록 하는 regression model
2) Shape to Attributes (S2A) : A2S와 반대로, shape(beta)에서 언어 속성의 score를 예측하도록 하는 regression model
3) Attributes & Measurements to Shape (AHWC2S) : 인체 측정값(chest, waist, hip 둘레, 키, 몸무게)에서 shape을 예측하도록 하는 regression model을 설계하고, 언어 속성도 같이 이용 가능하다면 이용한다.
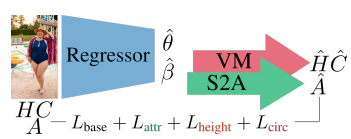
training details
ExPose의 network weight로 SHAPY를 초기화하고, Monocular expressive body regression through body-driven attention, H3.6M, SPIN 학습 데이터 및 모델 에이전시 데이터셋을 사용한다.
각 배치의 50% 이미지는 모델 에이전시 이미지에서 샘플링하도록 하여, 어느 정도의 성별 균형을 보장하게 만든다.
1-3) OSSO: Obtaining Skeletal Shape from Outside
OSSO는 SMPL body(ply)를 input으로 넣으면, 실제 뼈와 같은 형태로 시각화해준다.
시각화한 결과는 ply 파일(3D)로 저장된다.
input으로 ply 파일이 사용되는데, 보통 모델들이 출력으로 사용하는 obj 형식을 ply로 변환하면 된다.
변환 파이썬 코드는 이 코드를 참고해도 된다.
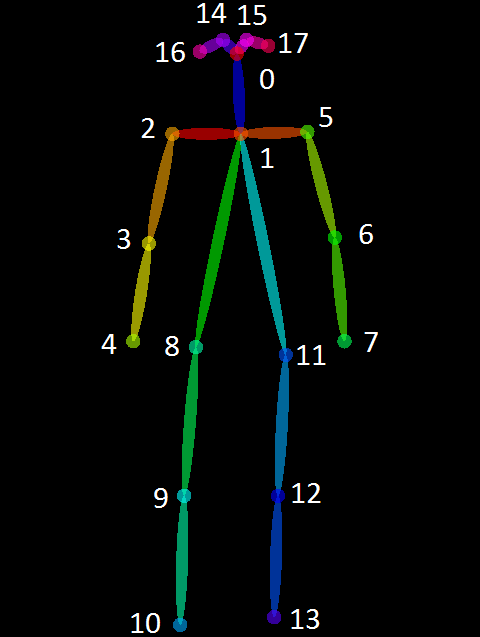
단순히 joint에 대한 20여개의 점들로만 표현되는 기존의 방식에 비해, 시각화를 더 현실적으로 할 수 있다.
OSSO는 실제 데이터를 활용한, 3D 신체 표면(Body Mesh)에서 내부 골격으로의 매핑을 학습한 최초의 시스템입니다.
데이터셋은 1000명의 남성, 1000명의 여성의 dual-energy X-ray 흡수 측정법(DXA) 스캔 데이터를 이용합니다.
DXA 데이터는 뼈 정보는 얻을 수 있지만, 3D Data를 만들지는 않기 때문에, STAR를 DXA 뼈 데이터에 fitting하는 형식으로 이용합니다.
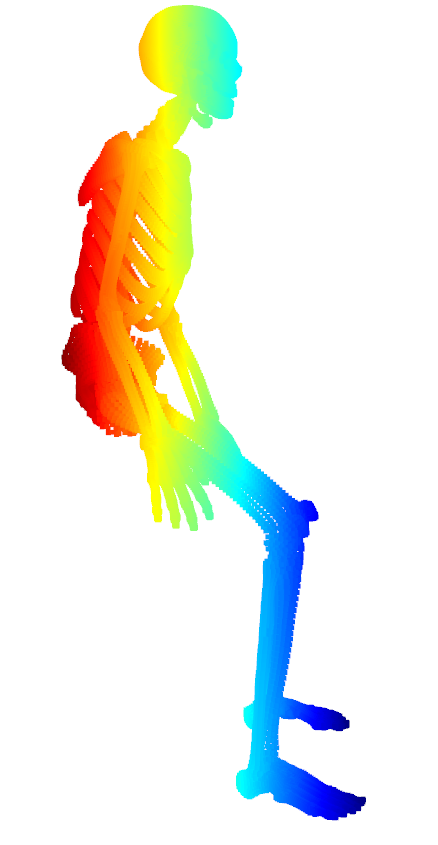
실제 추론을 해봐도, 꽤나 사실적인 결과가 나오는 걸 확인할 수 있습니다.
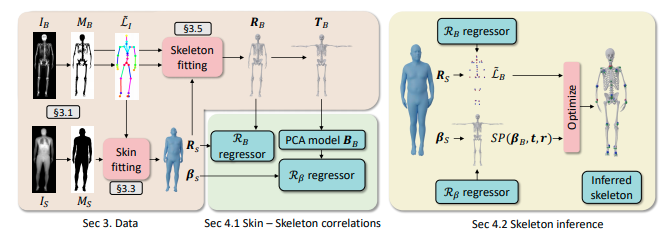
해당 논문의 Conclusion을 보면, 한계점으로 "극도로 마른 체형, 극도로 비만한 척추측만증, 노인, 어린이, 신체 일부가 없는 장애인" 등의 일부 경우를 지적하고 있습니다.
이런 점들은 이러한 모델을 만들 때 어쩔 수 없는 부분이라고 생각됩니다.
1-4) Putting people in their place: Monocular regression of 3d people in depth
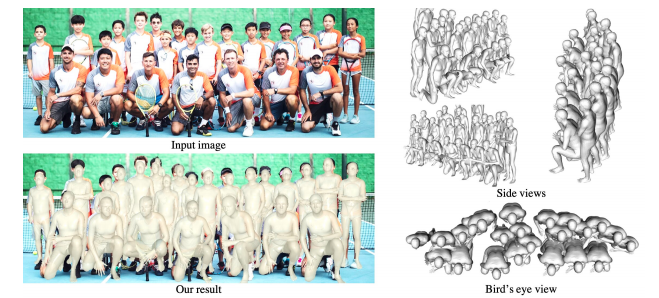
ROMP에서 발전된 모델로, 좀 더 depth와 multi people에 초점을 맞춘 논문입니다.
코드를 보면, pip 모듈로도 제공하고 있어서 편하게 설치하고 추론할 수 있습니다.

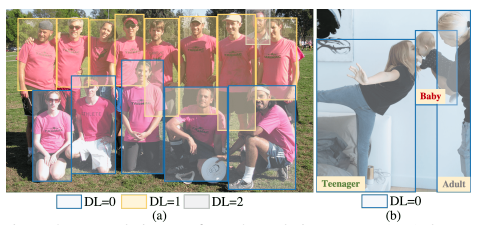
Bird's-eye-view(위에서 보는 뷰)를 통해 깊이 추정을 좀 더 잘 할 수 있도록 만들었고,
새로 제안하는 Related Human 데이터셋을 통해, Baby, Teenager, Adult 3가지로 shape을 분류하고, 상대적인 depth를 활용할 수 있도록 했습니다.
영유아 전용 body model인 SMIL과 SMPL을 같이 써서 baby도 모델링할 수 있게 했습니다.
이를 SMPL+A 모델이라 지칭하고(공식적인 모델보다는 이 논문에서 제안한 것 같습니다.), 연령 오프셋 α를 이용해, 성인 SMPL 템플릿 T와, 영유아 SMIL 템플릿 T1 사이를 보간하도록 합니다.
2. Whole Body
2-1) Embodied hands: Modeling and capturing hands and bodies together
2-2) Capturing and inferring dense full-body human-scene contact
3. Hand
3-1) Articulated Objects in Free-form Hand Interaction
3-2) GOAL: Generating 4D Whole-Body Motion for Hand-Object Grasping
4. Head
4-1) Im avatar: Implicit morphable head avatars from videos
5. Face
5-1) The neural coding of face and body orientation in occipitotemporal cortex
5-2) EMOCA: Emotion Driven Monocular Face Capture and Animation
6. 기타
6-1) TEMOS: Generating diverse human motions from textual descriptions
6-2) LocATe: End-to-end Localization of Actions in 3D with Transformers
6-3) BARC: Learning to Regress 3D Dog Shape from Images by Exploiting Breed Information
6-4) Human-aware object placement for visual environment reconstruction
'딥러닝, 머신러닝 > Pose Estimation' 카테고리의 다른 글
Azure Kinect camera calibration 환경 세팅 (0) | 2022.08.17 |
---|---|
depth prediction을 이용한 3d human pose estimation (0) | 2022.05.30 |
Whole Body pose estimation (0) | 2022.05.27 |
video 3D pose estimation (0) | 2022.05.26 |
Multi Person 3D Pose Estimation (0) | 2022.05.22 |
소중한 공감 감사합니다